How Hospitals Can Prevent Supply Shortages
Machine learning and predictive analytics take the guesswork out of supply forecasting
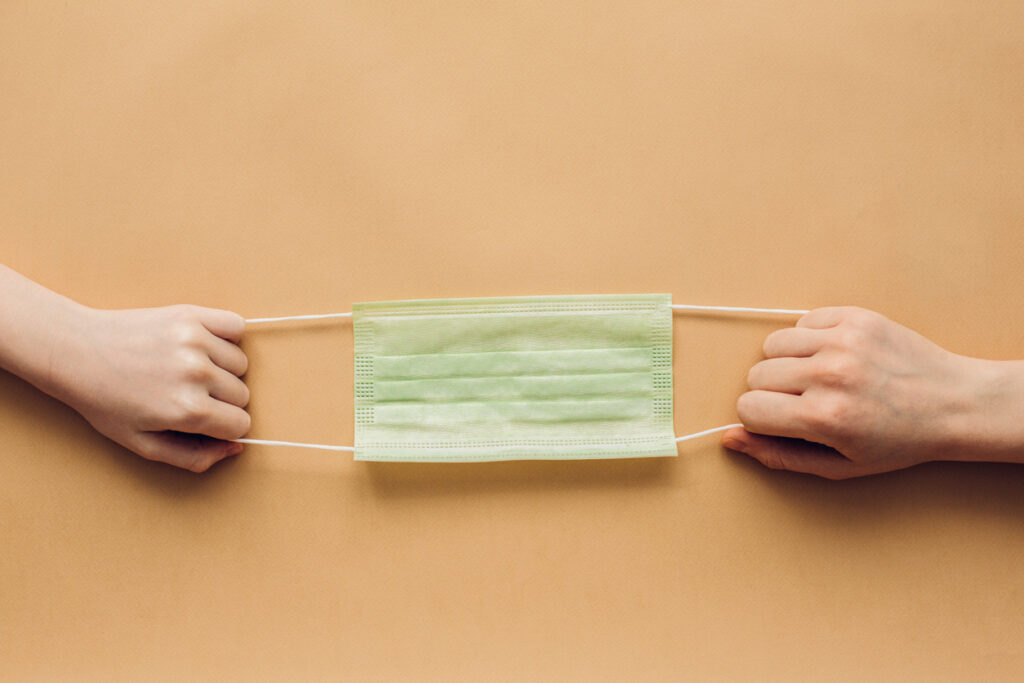
While the coronavirus pandemic was a crisis in and of itself, it was compounded by a severe shortage of personal protective equipment or PPE. Experts have called the PPE shortage of 2020 “an eventuality that nonetheless came as a surprise.” Healthcare workers, meanwhile, compared themselves to soldiers going into battle with cardboard for body armor. Nurses were forced to reuse disposable masks and respirators, while gloves, eye protection, and gowns were also in short supply.
Quality hospital care is always predicated on having the proper supplies and sufficient staff. Without the former, it’s more difficult to have the latter. Healthcare workers who lack PPE are more likely to become ill themselves, exasperating staffing shortages. Low counts of everything from ventilators to hospital meals can arise as the result of demographic shifts (i.e. the influx of older patients that is likely as Baby Boomers age) and one-off surges as well.
Today, for instance, hospital surges are due to the combination of COVID, the flu, and other respiratory viruses like RSV. Hospitals will need more supplies, from PPE to pharmaceuticals like antibiotics or aspirin—especially given current medication constraints. To prevent supply shortages, they need to be able to model their supply chain ahead of time. Advance planning is particularly important considering the majority of PPE must be imported from overseas.
The trouble with old-school methods
Accurate supply chain forecasting is dependent on having accurate forecasts of the patient population, including patient type, and the caregiver population. Every supply in the hospital can be linked to an individual patient or caregiver. For instance, a hospital may need one mask per caregiver or one ventilator for every five patients.
Planning the proper ratio of supplies to caregivers and patients requires thinking ahead at least six months to a year. But far too often, when hospitals plan, they are simply buying supplies based on past years’ needs, instead of trying to predict future ratios.
To make sure they are using the latest ratios and forecasts, hospitals, and integrated delivery networks (IDNs) should use machine learning and predictive analytics. Predictive analytics is a form of AI that continually takes raw, historical data and applies it to machine learning algorithms.
Machine learning and predictive analytics have many benefits, starting with the fact that they can analyze data at a far more granular level. Machine learning can consider more variables, such as seasonal ebbs and flows (like holidays) and external influences (including unexpected pandemics and natural disasters), to calculate both population forecasts and supply ratios.
The more information the system ingests, the smarter it becomes—and the more accurate the forecasts it provides. While understocking supplies can weigh on care, overstocking supplies is wasteful and costly. Granular machine learning-generated predictions offer far greater precision.
One Hospital’s Experience
The benefits of predictive analytics and machine learning are best illustrated through a recent real-life scenario.
When the pandemic first hit in early 2020, most hospitals were short on space and supplies—but children’s hospitals were not. This allowed one of BigBear AI’s customers—a 400+ bed children’s hospital–to take in other hospitals’ patient overflow. The hospital used predictive analytics and machine learning to accurately plan capacity—and supply needs—during the surge. The hospital was part of an interconnected network of regional healthcare facilities, which meant it could share and access census data with other in-network hospitals.
By combining anonymized information from past patients’ electronic health records with census data, the hospital was able to conduct a what-if scenario analysis on supply levels. The hospital ran several scenarios based on patient demographic information, case mix, and more, allowing it to only take on patients with the proper supplies to care for.
Using Digital Twins and Discrete-event Simulation to Predict Supplies
Digital twins—virtual replicas of the hospital or components of its facility—can take what-if scenario analysis to the next level. WISA and discrete-event simulation (DES) can be modeled in the digital twin, allowing decision-makers to answer questions like:
- Are we over-stocked or under-stocked on any particular supply?
- What if we have a 15% jump in our census? What supplies will we need to meet that surge?
- How long are patients staying in the hospital and how does that affect our supply needs?
By modeling various surges in a digital twin, hospital administrators—like those in the children’s hospital–can ensure they are prepared to handle the best, worst, and anything in between scenarios.
The bottom line
The pandemic was terrible, but it was also a teaching moment. Without the right supplies, it’s nearly impossible for hospitals to provide adequate care. Hospitals and IDNs must walk the fine line of stocking supplies for the most likely scenario, while also preparing for more extreme surges. Without the right technology, this is an extremely difficult task. The good news is that machine learning and predictive analytics offer more accurate supply chain forecasting while getting smarter over time.
Learn more
Learn more about major trends impacting supply chain forecasts. Download our whitepapers:
- Preparing for a Twindemic: Resource Planning in the Face of a COVID-19 and Flu Wave–and the Challenges to Getting it Right
- Preparing for the Baby Boom: Are Hospitals Ready to Meet the Needs of the Largest Demographic Entering the Healthcare System?
- How to Right-size Hospital Staff in the Face of Nursing Shortages
To learn more about BigBear.ai’s capacity planning solutions visit FutureFlowRX.