BigBear.ai’s Award-Winning Approach in Vision AI for Autonomous Defense Systems
In today’s world, no matter the environment or endeavor, it has become imperative that the Department of Defense – and all businesses alike – expect the unexpected. At BigBear.ai, we recognize that our AI systems need to be agile, adaptable, and ever-evolving. This reality was a central focus of the recent Autonomy 3 Ways Challenge, hosted by NIWC Atlantic Palmeto Tech Bridge. As a frontrunner in AI-driven solutions, BigBear.ai is proud to announce that we were awarded top honor in the competition.
The NIWC Atlantic PTB challenged AI innovators with three distinct problem areas: Computer Vision Surprise, Risky Facility Location, and Multi-Sensor Fusion for Autonomous Driving. Participants’ first milestone required a concise one-page submission detailing their strategies. Phase 2 spotlighted up to eight finalists, offering them the stage for in-person presentations in Myrtle Beach, SC. Orchestrated under the Naval Information Warfare Systems Command (NAVWAR) and embedded within the Department of Defense framework, this challenge aimed to further the U.S. Military’s goals for innovation in defense technology.
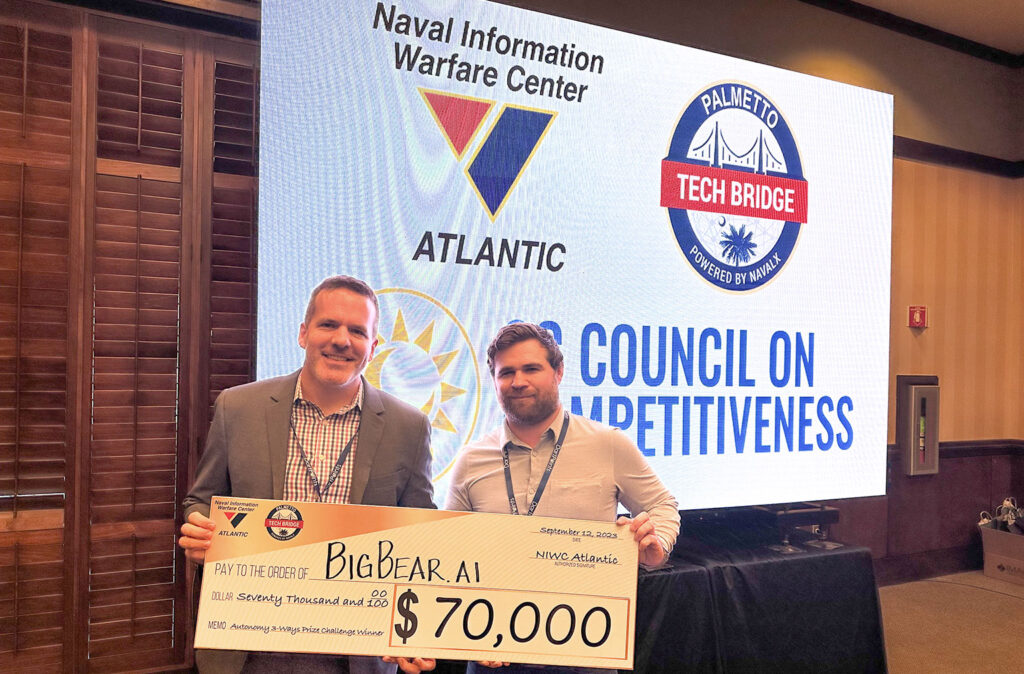
BigBear.ai’s Chris Morris (Left) and Ian Newell (Right) accept first place award.
Decoding the Challenge: BigBear.ai’s Innovations in Computer Vision
Our crowning achievement was attributed to a nuanced approach to the Computer Vision Surprise Challenge. Here, the task was ambitious: design AI methods to spot and categorize objects in ever-changing environments. From adapting to unique backdrops and integrating novel camera systems to identifying rare object classes and mitigating adversarial AI attacks, the demands were immense.
Instead of tethering to conventional methods, BigBear.ai synthesized several contemporary computer vision (CV) techniques into a unified system, ensuring adaptability to a myriad of complex, unexpected situations.
- Panoptic Segmentation and Object Similarity: By utilizing cutting-edge panoptic segmentation, our system could discern and segment objects even without prior knowledge of their classes. Coupled with object similarity models, our system assessed whether objects matched existing examples or introduced new classes. This duality enabled automatic discovery of new objects of interest and facilitated the training of supervised models to recognize them.
- Expanding Data Horizons: Using 3D models, synthetic examples were generated with automated data labeling. This approach allowed the system to imitate new conditions, whether they’re environmental or device-centric. Techniques like background replacement, gradient-based perturbation attacks, and random occlusion patterns ensured the robustness of the supervised model against adversarial interference.
Recognition and Beyond
Our novel techniques underwent a two-phase evaluation before being showcased at SC Decoded 2023 in Myrtle Beach, SC, earning acclaim from industry peers. “Our success at the Autonomy Challenge further demonstrates BigBear.ai’s resolve in harnessing AI for real-world challenges,” remarked Greg Goldwater, Chief Growth Officer at BigBear.ai. “Our approach not only meets but also anticipates the ever-evolving challenges in computer vision.”
To dive deeper into our groundbreaking autonomous systems solutions and witness firsthand our pioneering strides in AI-driven innovations, explore our autonomous systems solutions or email [email protected].